Blog
AI - A Ghost in the Machine?
An opinion piece from Daniel Gore, Product Manager of Thrive.App.
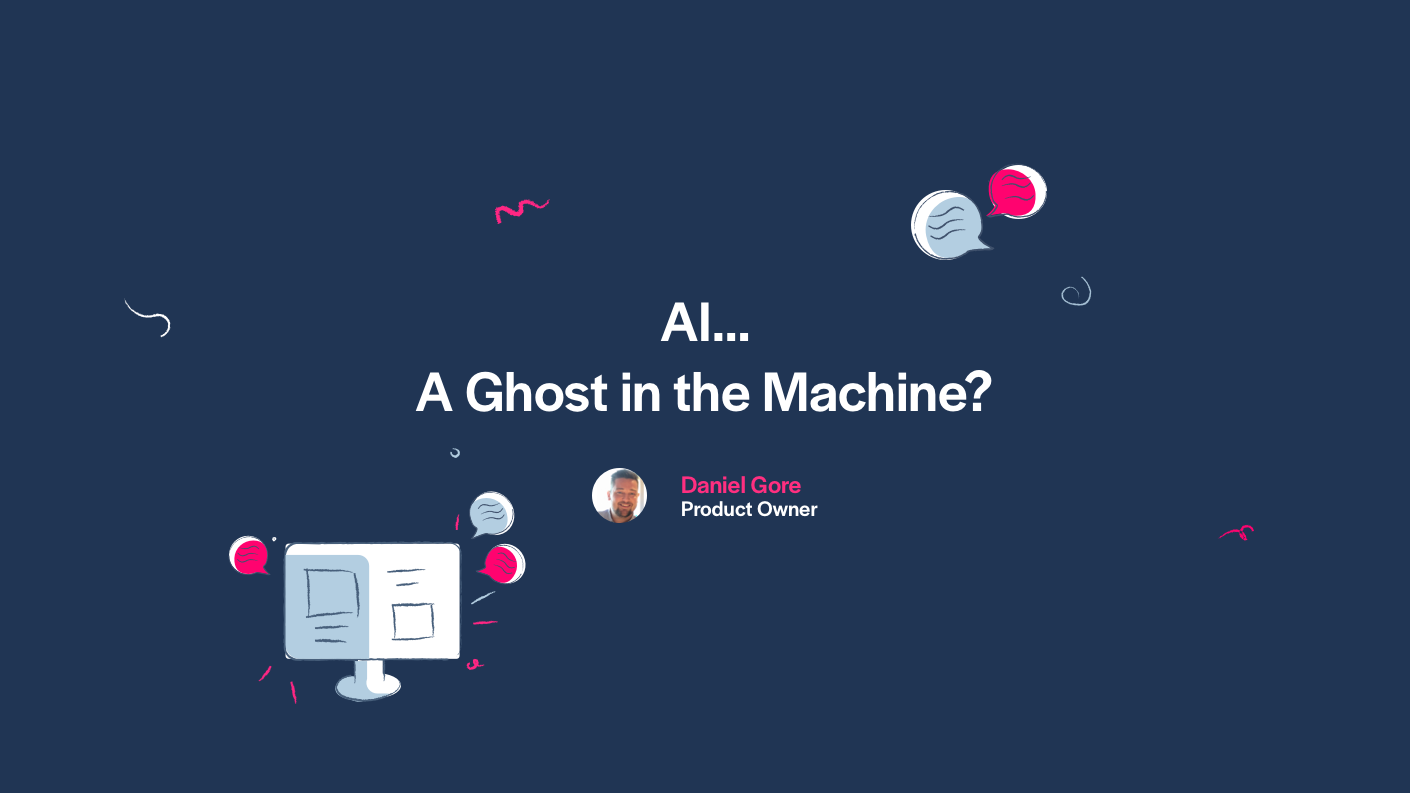
With the rise of Chat GPT, the hot question in town for any product manager, as the ubiquity of AI takes hold and the consumer perspective shifts, if you don’t have AI, does your software even work?
Alongside Chat GPT, we’ve also got the use of ‘Bard’ from Google and Microsoft’s ‘Copilot’. We’ve got numerous voice assistants and virtual assistants (that have been around for sometime) such as; Alexa, Siri, Cortana and Bixby. We’ve got writing assistants like Jasper, Hemmingway, Grammarly, Writely AI and Writesonic to name just a few. There are AI video generators like Pictory, Synthesia, Deepbrain AI and Wave Video, and we’ve got productivity assistants like Calendly, Krisp, sales and marketing AI tools like HubSpot, Flick and Growbar, we’ve got transcription software and everything else in between, the list is endless. You can also get your car to drive you to work with AI or get robots to clean your home with ‘AI backed obstacle avoidance’ tooling. And just in case none of these suit, there are weekly releases of all new tools - what an age to be alive.
The phrase a ‘ghost in the machine’ descends from a philosophical critique developed in 1949 by British philosopher Gilbert Ryle. He used it to counter the mind-body argument within the field of cognitive science put forward by René Descartes. The phrase has also been used to describe the emergence of AI and therefore provides a vaguely original title for this piece. In its most basic form, the mind-body thesis, is that the mind (broadly speaking ‘consciousness’) and the body are separate entities. Descartes famously said ‘I think therefore I am’ and posited the idea that the mind is non-physical, with the body being physical. Ryle countered by describing that mental processes and consciousness are intricately intertwined with the functioning of the body, there isn’t a ‘ghost in the machine’ that simply inhabits the body and controls it. Another, way to think about this is to borrow the ‘Zombie argument’ by David Chalmers. A zombie can function without any kind of ‘consciousness’. A zombie walks, groans and tries to eat people but it doesn’t ‘think’.
Is AI simply this, just ghosts and zombies within our internet machine? If these tools are just following and extrapolating a set of rules and principles, taking inputs and actioning outputs, do these tools really ‘think’? Or do the ghosts just crank the machine much faster and more accurately than we ever could, creating the illusion of ‘thinking’?
I asked Chat GPT to describe itself. It helpfully agreed with my assumption that at its core, it is a well resourced and complex ‘next word predictor’. GPT stands for Generative Pre-trained Transformer. It is a type of language model designed to generate human-like text by predicting the next word in a sequence based on the context provided by the words that come before it (as well as the mountains of information it has been trained on). We’ve seen this before. We’ve all experienced some use of language models in our daily lives already. The natural language processing and computational linguistics of Alexa and Siri respond to questions to give responses, Grammarly interprets written text to check for errors leveraging neural networks and algorithms to provide grammatical corrections and improve text fluency. A multitude of tools already use this ‘stuff’ in some form or another. So why all this fuss all of a sudden?
The Gartner Hype cycle also doesn’t overly help given the tsunami of terms, interpretations and divergence that exists. The Hype Cycle consists of five main phases:
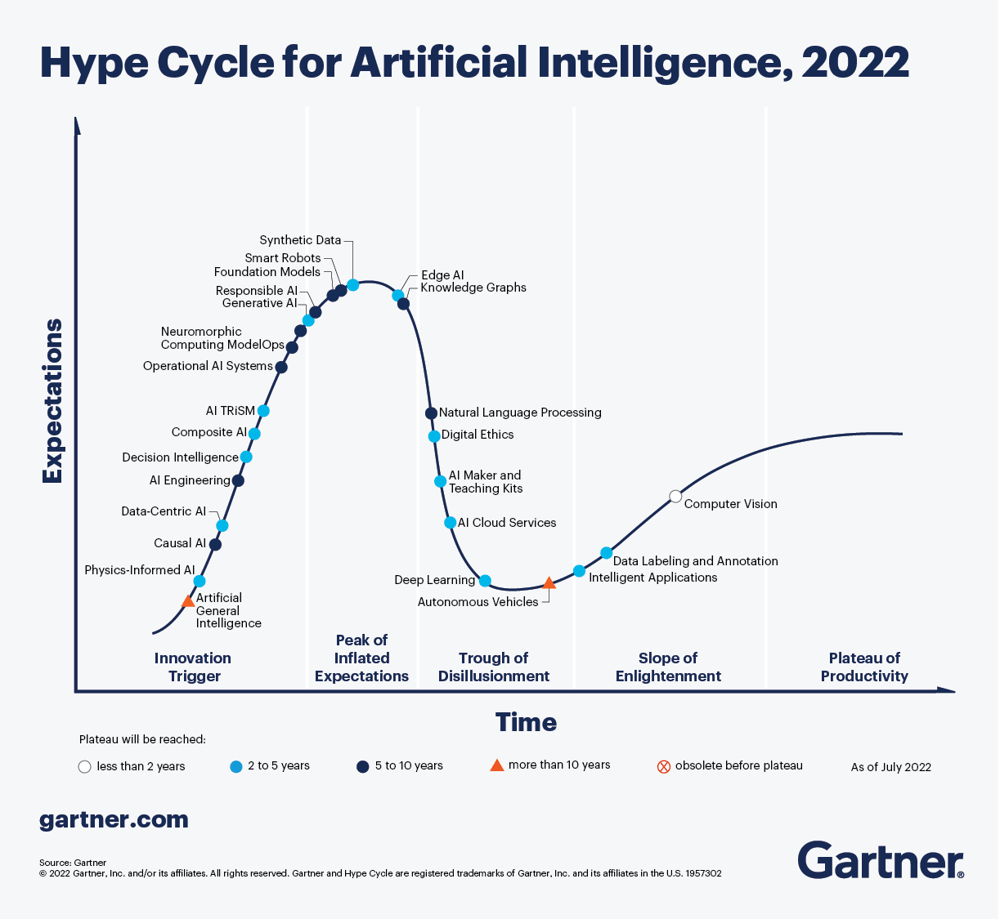
- Technology Trigger: This is the initial stage when a new technology or trend is introduced or becomes more widely known. It may be driven by breakthroughs, media attention, or early proof-of-concept demonstrations.
- Peak of Inflated Expectations: In this phase, excitement and expectations surrounding the technology reach their highest point. There is often a significant amount of hype, media coverage, and inflated optimism about the potential benefits and possibilities of the technology. However, there may be limited practical applications or tangible results at this stage.
- Trough of Disillusionment: As the initial hype subsides and reality sets in, the technology enters a phase of disillusionment. This stage is characterised by a decline in interest and a realisation of the limitations, challenges, or overhyped expectations associated with the technology. Some early adopters may face difficulties or setbacks during this phase.
- Slope of Enlightenment: During this phase, a more balanced and pragmatic understanding of the technology emerges. Lessons are learned from the challenges faced during the previous stage, and the technology's true value and potential become clearer. Organisations and individuals start to identify and adopt more practical and sustainable use cases for the technology.
- Plateau of Productivity: This is the final phase where the technology reaches a state of maturity and widespread adoption. It becomes more stable, standardised, and integrated into various industries and applications. The technology's benefits and value are more widely recognised and realised, leading to broader and more practical usage.
Where is Chat GPT on the graph? Broadly speaking, Chat GPT could be classified as a form of natural language processing, leveraging transformer algorithms (a type of neural network) to provide human-like responses. Natural Language Processing currently sits within the trough of disillusionment and therefore the cycle indicates that we’ve already been through the initial hype and we’ve past the peak of inflated expectations. Why has Chat GPT suddenly eclipsed all of the previous attempts to market AI text prediction? There is meant to be a subsiding of the hype at this stage, but Chat GPT has dominated (and in some areas continues) to dominate the discussion.
The answer may lie in the implementation rather than the technology. As with many things ‘product,’ it isn’t necessarily what it does, it's how it does it. Perhaps our previous experiences with Alexa and Siri have conditioned us to know that the responses are limited, that we have to be clear with our instructions and we almost know the limits of our requests before we’ve asked any questions. A good example of this low bar might be the ‘help’ sections on websites that use chatbots to give us half-baked answers only for us to inevitably wait until we can click the button that lets us ‘talk to an agent’. In this scenario, responses often feel pre-programmed, it feels like we are just being funnelled down a script. The icing on the cake is when we are asked ‘Is this correct?’ or ‘Did this help?’ casting doubt over the entire validity of the machine’s aptitude and existence.
Interestingly enough this kind of shared experience (any shared experience) also plays a massive role in cultural development, arguably making its way through to market expectations. If we take this principle forward, perhaps the hype graph is correct, maybe we’ve all seen these ‘AI tools’, used them, tested them, had some enjoyment and seen some limited use or marginal success but ultimately we’ve got bored and moved on.
However, when Chat GPT was introduced, it didn’t behave like the things we’ve used before. Individuals could just talk to it. Chat GPT gave well-rounded and well-formatted answers, took criticism and instruction, managed sarcasm, handled idioms, had a ludicrous range of knowledge retrieval, picked up on language nuances, happily overcame any end-user typographical errors, told jokes, wrote poetry, could translate text, generated code snippets, could simplify, rewrite and summarise its own answers, uses sentiment analysis and conversational abilities to maintain context and coherence throughout a prolonged conversation and all of this combined ultimately created the illusion or feel of ‘natural’ conversation - it ‘sounded’ like someone. Even to the point where the UI would pause after you entered a question. The slight pause, be that internet connection latency or intentional UI/UX, sometimes made it feel like it was ‘thinking’ before answering.
The experience felt entirely different to anything that the average consumer had experienced before. Perhaps then it is simply the combination and the assembly of this tool that has generated such hype. Finally, we may well have an educated zombie in the machine or even a friendly ghost.
Regardless of whether you interact with AI tools in your day-to-day life, regardless of the current capabilities or the future potential of AI, has the media exposure of Chat GPT totally shifted market expectations within software. Could it be that this shift now requires your product to have AI soon enough, or risk becoming ‘old technology’. Is AI now becoming a ‘standard’ feature? The question for software product managers might not be if you should have AI, but when.
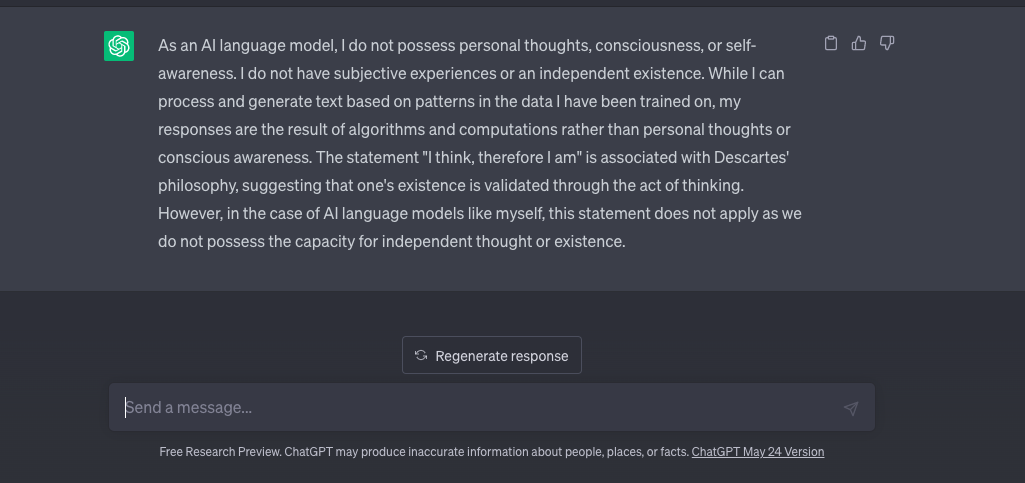
Final Thoughts
Is AI becoming a standard feature in software products? Are market expectations shifting due to the media exposure of Chat GPT? These are important questions for software product managers to consider, as the combination and assembly of AI tools like Chat GPT have generated a new level of hype and expectation. The experience of interacting with Chat GPT feels entirely different from anything consumers have experienced before, and this may be driving a need for AI in software products sooner rather than later.
Considering the impact of AI on software products, it's worth pondering what role it will play in Internal Comms and Employee Engagement in the future.
With the new standards being set by Chat GPT, it's important to consider what AI features could be incorporated into a Thrive employee app to keep up with the changing market expectations. So, what do you think would be a valuable addition to the app's AI capabilities?